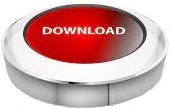

- WEIGHTED AVERAGE METHOD MAP HOW TO
- WEIGHTED AVERAGE METHOD MAP CODE
- WEIGHTED AVERAGE METHOD MAP FREE
f1_score_weighted: weighted mean by class frequency of F1 score for each class.f1_score_micro: computed by counting the total true positives, false negatives, and false positives.f1_score_macro: the arithmetic mean of F1 score for each class.However, it does not take true negatives into account. It is a good balanced measure of both false positives and false negatives. average_precision_score_binary, the value of average precision by treating one specific class as true class and combine all other classes as false class.īalanced accuracy is the arithmetic mean of recall for each class.į1 score is the harmonic mean of precision and recall.average_precision_score_weighted, the arithmetic mean of the average precision score for each class, weighted by the number of true instances in each class.average_precision_score_micro, computed by counting the total true positives, false negatives, and false positives.average_precision_score_macro, the arithmetic mean of the average precision score of each class.AUC_binary, the value of AUC by treating one specific class as true class and combine all other classes as false class.Īccuracy is the ratio of predictions that exactly match the true class labels.Īverage precision summarizes a precision-recall curve as the weighted mean of precisions achieved at each threshold, with the increase in recall from the previous threshold used as the weight.AUC_weighted, arithmetic mean of the score for each class, weighted by the number of true instances in each class.AUC_micro, computed by counting the total true positives, false negatives, and false positives.AUC_macro, the arithmetic mean of the AUC for each class.MetricĪUC is the Area under the Receiver Operating Characteristic Curve. Refer to image metrics section for additional details on metrics for image classification models. For more detail, see the scikit-learn documentation linked in the Calculation field of each metric. The following table summarizes the model performance metrics that automated ML calculates for each classification model generated for your experiment. Learn more about binary vs multiclass metrics in automated ML. If classes have different numbers of samples, it might be more informative to use a macro average where minority classes are given equal weighting to majority classes. While each averaging method has its benefits, one common consideration when selecting the appropriate method is class imbalance. Weighted - Calculate the metric for each class and take the weighted average based on the number of samples per class.Micro - Calculate the metric globally by counting the total true positives, false negatives, and false positives (independent of classes).Macro - Calculate the metric for each class and take the unweighted average.Scikit-learn provides several averaging methods, three of which automated ML exposes: macro, micro, and weighted. Many classification metrics are defined for binary classification on two classes, and require averaging over classes to produce one score for multi-class classification. These metrics are based on the scikit learn implementation. In the Metrics tab, use the checkboxes on the left to view metrics and charts.Īutomated ML calculates performance metrics for each classification model generated for your experiment.In the Models tab, select the Algorithm name for the model you want to evaluate.In the table at the bottom of the page, select an automated ML job.Select your experiment from the list of experiments.Sign into the studio and navigate to your workspace.
WEIGHTED AVERAGE METHOD MAP HOW TO
The following steps and video, show you how to view the run history and model evaluation metrics and charts in the studio:
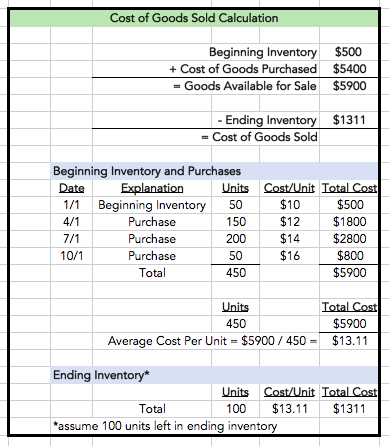
WEIGHTED AVERAGE METHOD MAP CODE
The Azure Machine Learning studio (no code required)Īfter your automated ML experiment completes, a history of the jobs can be found via:.An Azure Machine Learning experiment created with either:.
WEIGHTED AVERAGE METHOD MAP FREE
(If you don't have an Azure subscription, create a free account before you begin) Certain features might not be supported or might have constrained capabilities.įor more information, see Supplemental Terms of Use for Microsoft Azure Previews. The preview version is provided without a service level agreement, and it's not recommended for production workloads. Items marked (preview) in this article are currently in public preview.
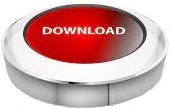